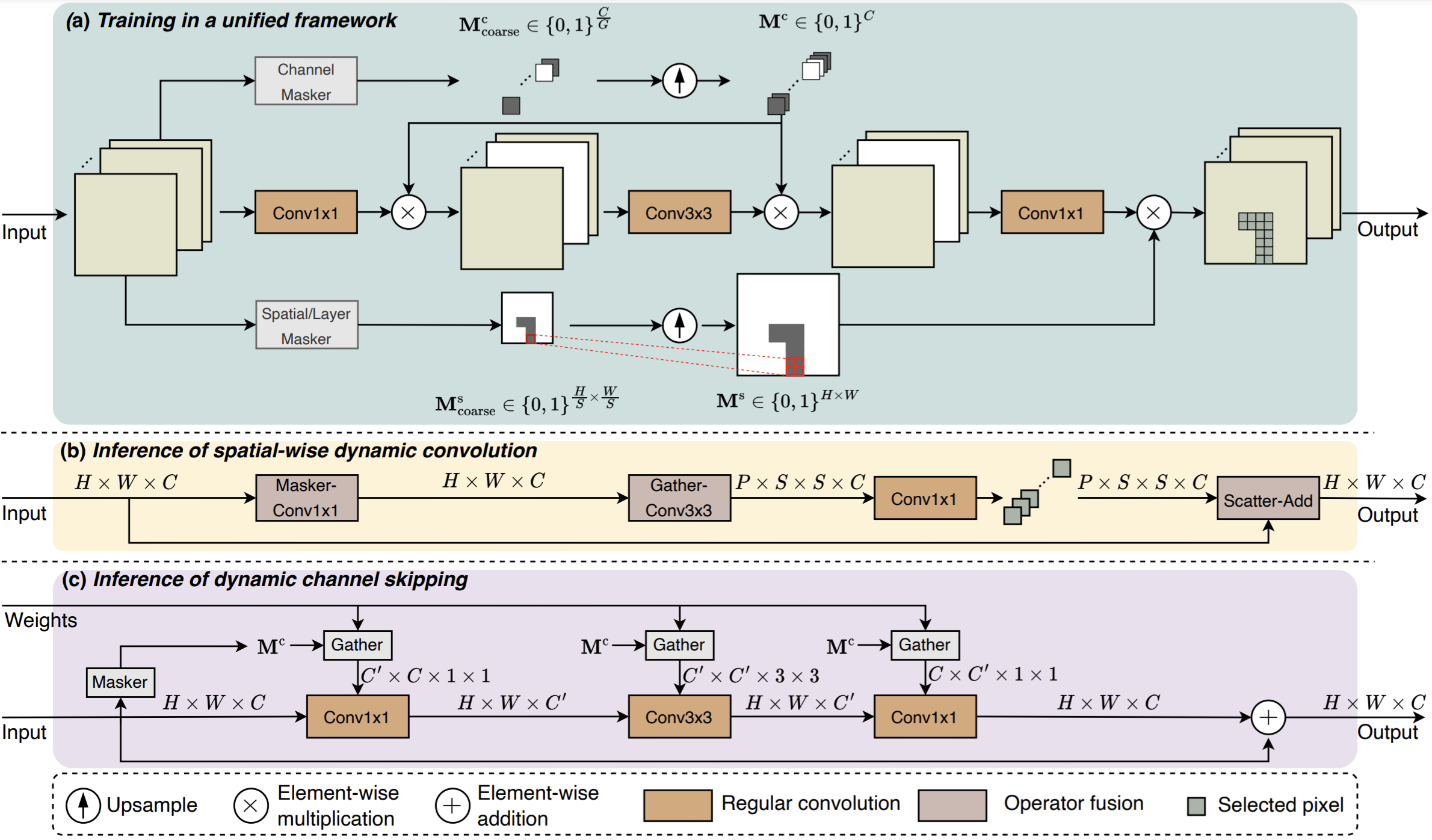
Latency-aware Unified Dynamic Networks for Efficient Image Recognition [PDF] [Code]
Yizeng Han*, Zeyu Liu*, Zhihang Yuan*, Yifan Pu, Chaofei Wang, Shiji Song, Gao Huang
IEEE Transactions on Pattern Analysis and Machine Intelligence (TPAMI, IF=24.314), 2024
We propose Latency-aware Unified Dynamic Networks (LAUDNet), a comprehensive framework that amalgamates three cornerstone dynamic paradigms—spatially-adaptive computation, dynamic layer skipping, and dynamic channel skipping—under a unified formulation.
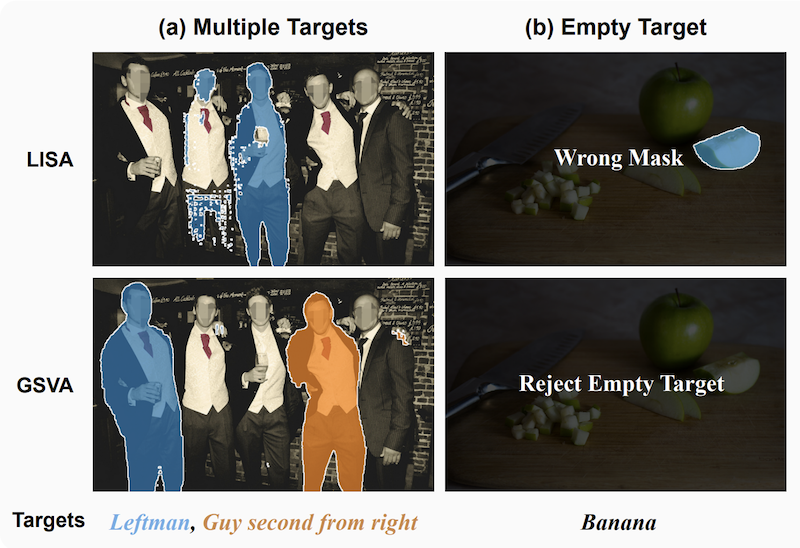
GSVA: Generalized Segmentation via Multimodal Large Language Models [PDF] [Code]
Zhuofan Xia, Dongchen Han, Yizeng Han, Xuran Pan, Shiji Song, Gao Huang
IEEE/CVF Conference on Computer Vision and Pattern Recognition (CVPR), 2024
We propose Generalized Segmentation Vision Assistant (GSVA) to address the issues of multi-object and empty-object in Generalized Referring Expression Segmentation (GRES).
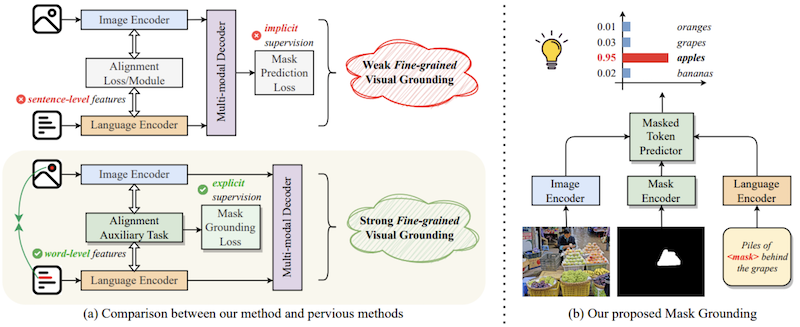
Mask Grounding for Referring Image Segmentation [PDF]
Yong Xien Chng, Henry Zheng, Yizeng Han, Xuchong Qiu, Gao Huang
IEEE/CVF Conference on Computer Vision and Pattern Recognition (CVPR), 2024
We introduce a novel Mask Grounding auxiliary task that significantly improves visual grounding within language features, by explicitly teaching the model to learn fine-grained correspondence between masked textual tokens and their matching visual objects.
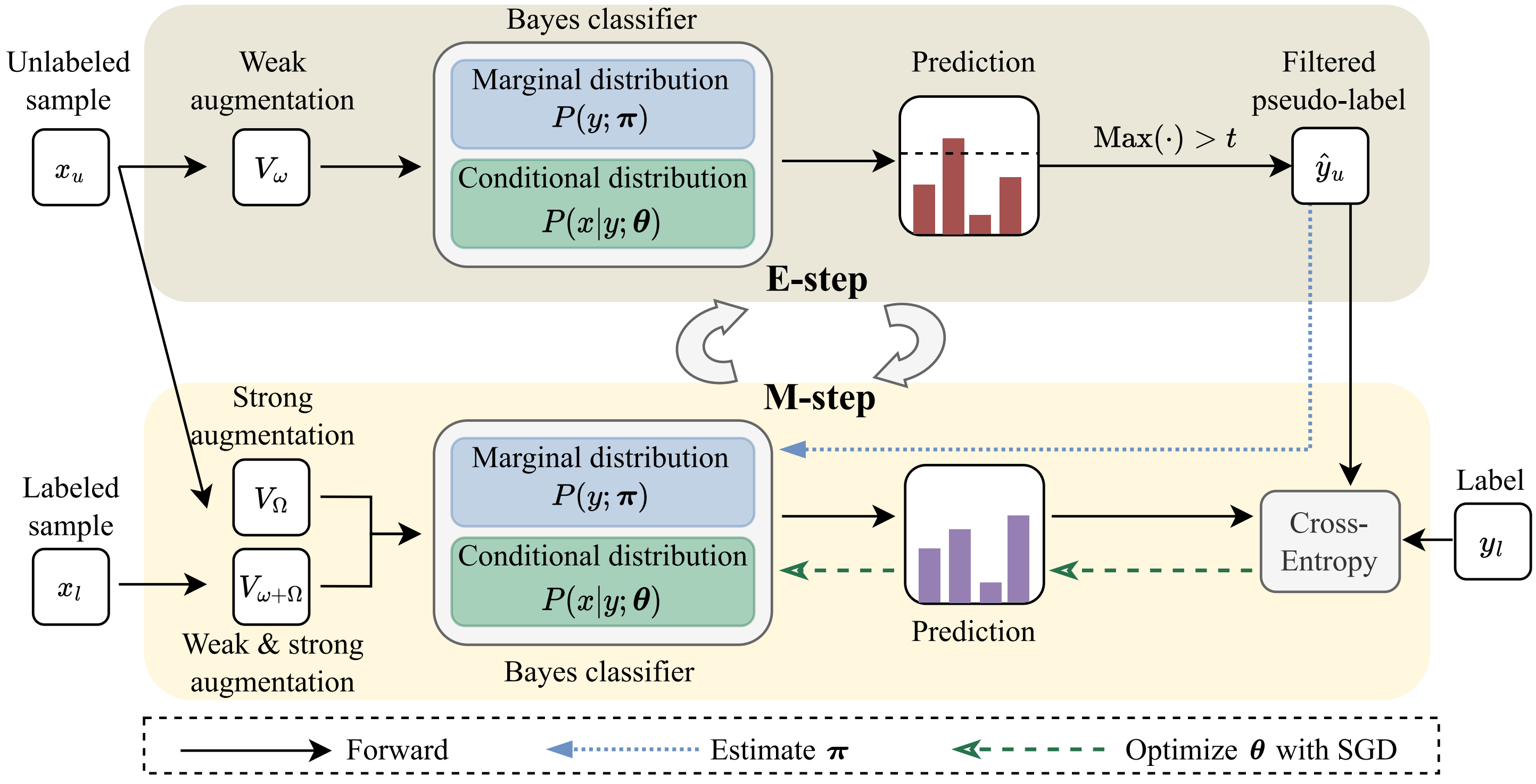
SimPro: A Simple Probabilistic Framework Towards Realistic Long-Tailed Semi-Supervised Learning [PDF] [Code]
Chaoqun Du*, Yizeng Han*, Gao Huang
Arxiv Preprint, 2024
We focus on a realistic yet challenging task: addressing imbalances in labeled data while the class distribution of unlabeled data is unknown and mismatched. The proposed SimPro does not rely on any predefined assumptions about the distribution of unlabeled data.
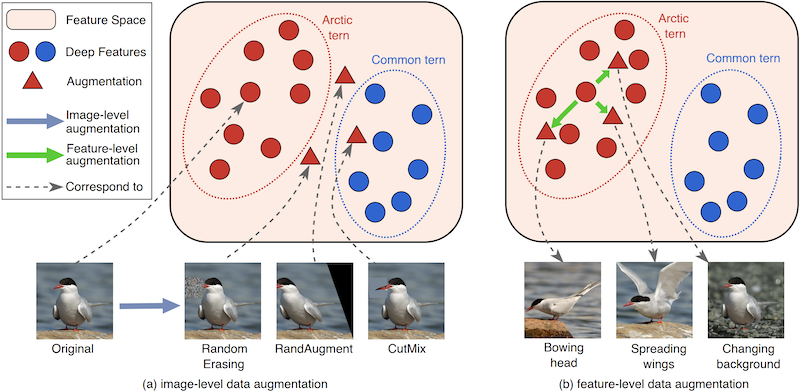
Fine-grained Recognition with Learnable Semantic Data Augmentation [PDF] [Code]
Yifan Pu*, Yizeng Han*, Yulin Wang, Junlan Feng, Chao Deng, Gao Huang
IEEE Transactions on Image Processing (TIP), 2023
We propose diversifying the training data at the feature space to alleviate the discriminative region loss problem in fine-grained image recognition. Specifically, we produce diversified augmented samples by translating image features along semantically meaningful directions. The semantic directions are estimated with a sample-wise covariance prediction network.
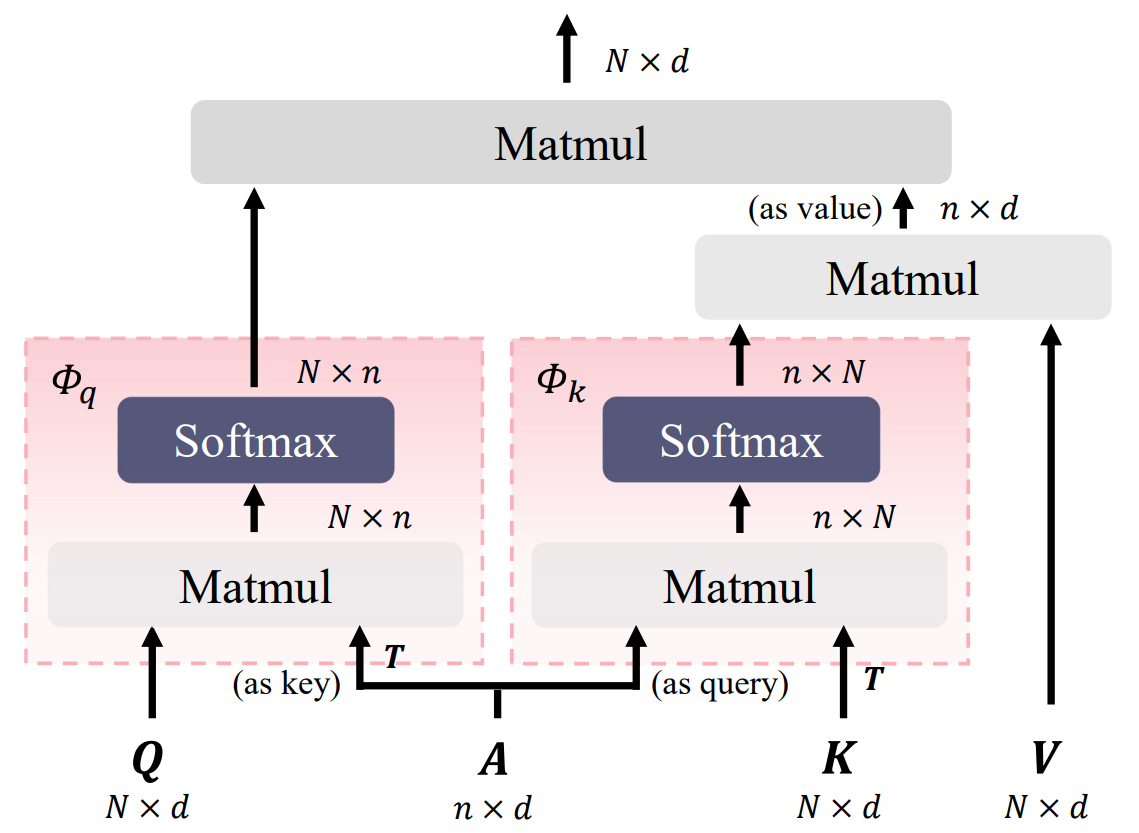
Agent Attention: On the Integration of Softmax and Linear Attention [PDF] [Code]
Dongchen Han, Tianzhu Ye, Yizeng Han, Zhuofan Xia, Shiji Song, Gao Huang
Arxiv Preprint, 2024
We propose Agent Attention, a linear attention mechanism in vision recognition and generation. Notably, agent attention has shown remarkable performance in high-resolution scenarios, owning to its linear attention nature.
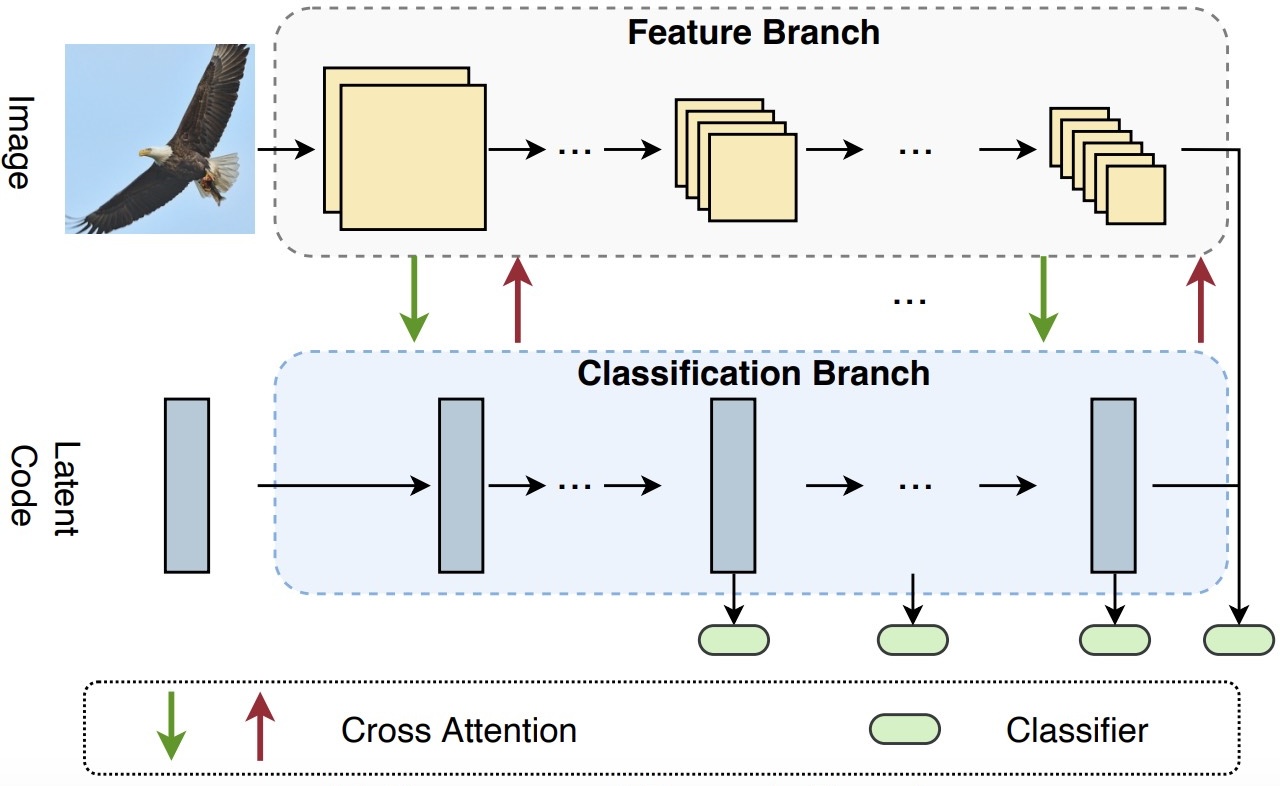
Dynamic Perceiver for Efficient Visual Recognition [PDF] [Code]
Yizeng Han*, Dongchen Han*, Zeyu Liu, Yulin Wang, Xuran Pan, Yifan Pu, Chao Deng, Junlan Feng, Shiji Song, Gao Huang
IEEE/CVF International Conference on Computer Vision (ICCV), 2023
We propose Dynamic Perceiver (Dyn-Perceiver), a general framework which can be conveniently implemented on top of any visual backbones. It explicitly decouples feature extraction and early classification. We show that early classifiers can be constructed in the classification branch without harming the performance of the last classifier. Experiments demonstrate that Dyn-Perceiver significantly outperforms existing state-of-the-art methods in terms of the trade-off between accuracy and efficiency.
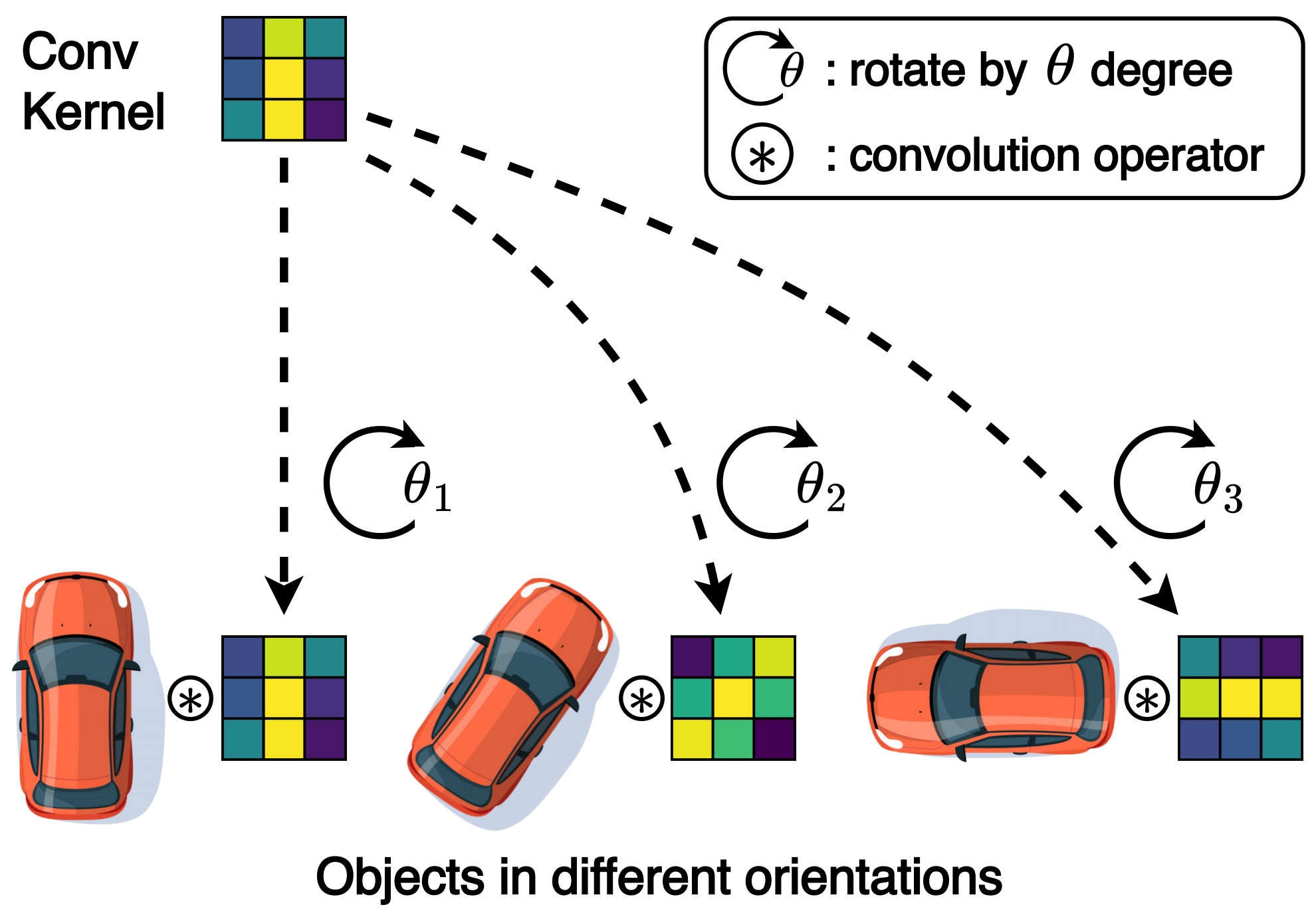
Adaptive Rotated Convolution for Rotated Object Detection [PDF] [Code]
Yifan Pu*, Yiru Wang*, Zhuofan Xia, Yizeng Han, Yulin Wang, Weihao Gan, Zidong Wang, Shiji Song, Gao Huang
IEEE/CVF International Conference on Computer Vision (ICCV), 2023
We propose adaptive rotated convolution (ARC) for rotated object detection. In the proposed approach, the convolution kernels rotate adaptively according to different object orientations in the images. The ARC module can be plugged into any backbone networks with convolution layer. Our work achievs SOTA performance on the DOTA benchmark.
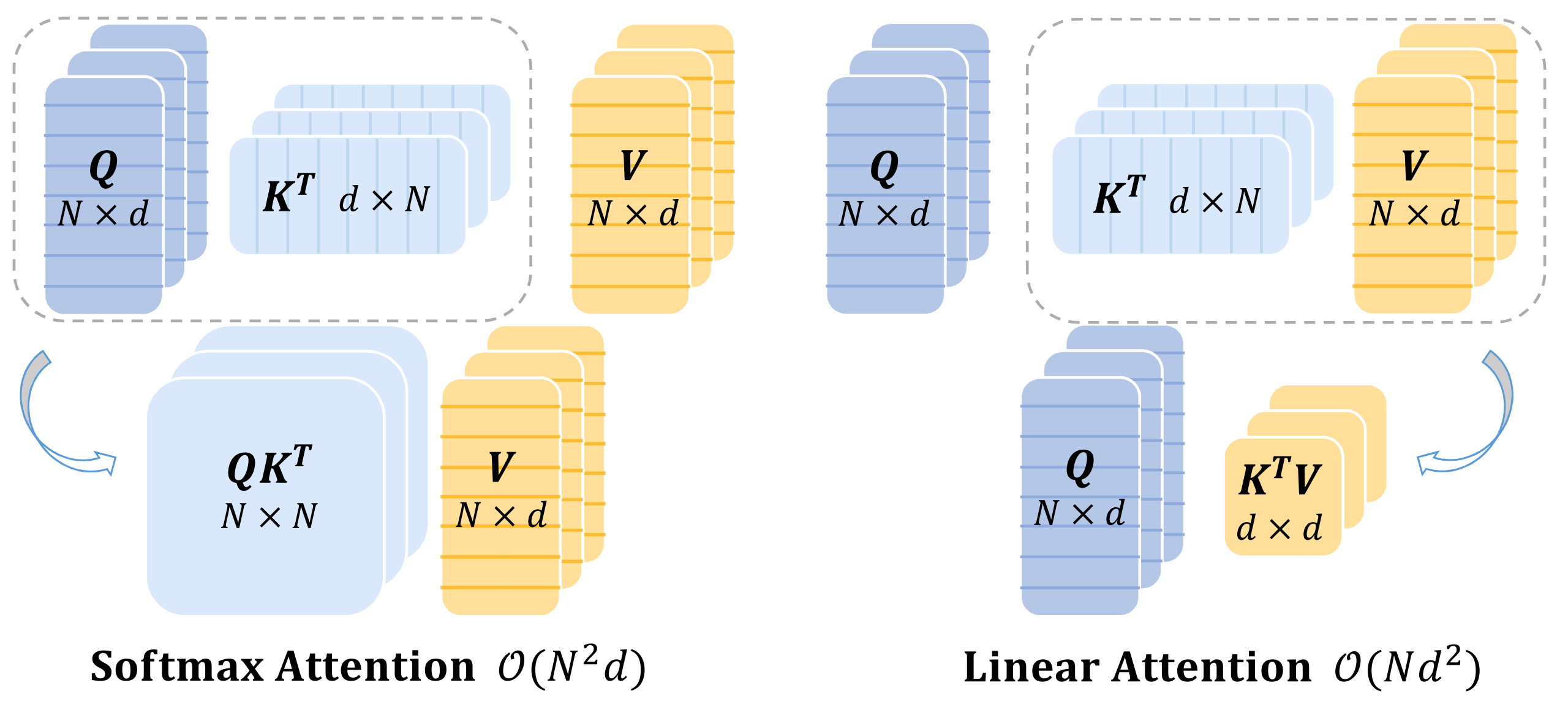
FLatten Transformer: Vision Transformer using Focused Linear Attention [PDF] [Code]
Dongchen Han*, Xuran Pan*, Yizeng Han, Shiji Song, Gao Huang
IEEE/CVF International Conference on Computer Vision (ICCV), 2023
We propose a novel focused linear attention module. By addressing the limitations of previous linear attention methods from focus ability and feature diversity perspectives, our module achieves an impressive combination of high efficiency and expressive capability.